1. 인공지능 확산에 따른 문제점
인터넷이나 스마트폰과 같은 신기술이 우리의 일상과 사회를 빠르고 편리하게 변화시킨 동시에 새로운 문제가 등장했듯, 사회의 많은 부분에 인공지능이 활용되면서 새로운 문제나 위험에 대한 우려가 등장했다. 이러한 문제 상당수는 사회적, 윤리적인 문제이므로 단순히 기술을 개선하거나 신기술을 도입하는 것만으로는 해결할 수 없다. 실제로 사용자가 인공지능 기술을 악의적인 목적으로 활용하거나 인공지능이 반사회 ・ 반인륜적인 판단을 하여 사회적 혼란을 유발하는 사건이 종종 발생하고 있다.
인공지능 사고사례
-
사고사례1: 챗GPT가 알려준 가짜 판례 제출한 美 변호사
미국의 한 변호사가 챗GPT가 제시한 판례가 담긴 의견서를 법원에 제출. 그러나 해당 판례는 모두 가짜인 것으로 드러났으며, 챗GPT에 '진짜 판례가 맞느냐'교 거듭 확인했으나, 끝까지 '그렇다고' 주장('23.5.)
- 시사점
- 생성형 인공지능의 할루시네이션Hallucination 발생에 따른 우려
-
사고사례 2: 가짜 인터뷰 기사를 만든 인공지능 챗봇
독일의 한 주간지는 인공지능 챗봇을 활용해 인터뷰 내용을 만들어 만난 적도 없는 유명 레이싱 선수의 가짜 인터뷰 기사를 대서 특필('23.4)
- 시사점
- 인공지능을 통해 가짜 정보를 제작 및 유포하여 사회적 혼란 유발
-
사고사례 3: 체스 경기 도중 사고 발생
모스크바 체스 토너먼트에서 체스 로봇과 경기를 벌이던 7세 소년이 안전 규정을 어겨 로봇에 의해 손가락이 부러짐('22.7.)
- 시사점
- 인공지능과 연동된 하드웨어로 인한 안전사고 발생
-
사고사례 4: 배달 플랫폼 배차 알고리즘 공개 요구
배달원들은 배달 플랫폼의 인공지능 알고리즘 배차가 노동 통제 및 불공정한 배달료 문제를 유발한다고 주장('21.6.)
- 시사점
- 인공지능의 추론 결과에 대한 사용자의 문제 제기
2. 인공지능 신뢰성 개념
앞서 사례에서 살펴봤듯 인공지능 제품・서비스는 단지 ‘구현할 수 있는가?’라는 기술적 측면뿐 아니라 ‘이 제품・서비스가 존재해도 괜찮은가?’라는 윤리적 측면에서도 검토해야 한다. 특히 인공지능이 다양한 분야에 활용되면서 인공지능 시스템과 학습 모델에 윤리적인 결함이 있는데도 이를 인지하지 못한 채 사용될 경우 매우 큰 파급효과를 낳을 수 있다. '인공지능 신뢰성'이란 데이터 및 모델의 편향, 인공지능 기술에 내재한 위험과 한계를 해결하고, 인공지능을 활용하고 확산하는 과정에서 부작용을 방지하기 위해 준수해야 하는 가치 기준을 말한다. 주요 국제 기구를 중심으로 인공지능 신뢰성을 확보하는 데 필수적인 요소가 무엇인지 활발한 논의가 이루어지고 있다. 일반적으로 안전성, 설명가능성, 투명성, 견고성, 공평성 등이 신뢰성을 확보하는 데 필수적인 요소로 거론되고 있다.
인공지능 신뢰성의 주요 핵심 속성 및 의미
핵심속성 | 의미 |
---|---|
안전성safety | 인공지능이 판단・예측한 결과로 시스템이 동작하거나 기능이 수행됐을 때 사람과 환경에 위험을 줄 가능성이 완화 또는 제거된 상태 |
설명가능성explainability | 인공지능의 판단・예측의 근거와 결과에 이르는 과정이 사람이 이해할 수 있는 방식으로 제시되거나, 문제 발생 시 문제에 이르게 한 원인을 추적할 수 있는 상태 |
투명성transparency | 인공지능이 내리는 결정에 대한 이유가 설명 가능하거나 근거가 추적 가능하고, 인공지능의 목적과 한계에 대한 정보가 적합한 방식으로 사용자에게 전달되는 상태 |
견고성robustness | 인공지능이 외부의 간섭이나 극한적인 운영 환경 등에서도 사용자가 의도한 수준의 성능 및 기능을 유지하는 상태 |
공평성fairness | 인공지능이 데이터를 처리하는 과정에서 특정 그룹에 대한 차별이나 편향성을 나타내거나, 차별 및 편향을 포함한 결론에 이르지 않는 상태 |
※ 프라이버시privacy, 지속가능성sustainability 등도 핵심 속성 중 하나로서 다양하게 논의 중
- Caution주요 기관에서 논의 중인 인공지능 신뢰성 개념
- (국제표준화기구, ISO) 신뢰성의 세부 속성으로 가용성availability, 회복탄력성resiliency, 보안성security, 프라이버시, 안전성, 책임성, 투명성, 통합성integrity 등 제시(ISO/IEC TR 24028: '20)
- (경제협력개발기구, OECD) 지속가능한 사회와 인간 중심의 가치에 부합하고 투명성, 설명가능성, 견고성 및 안전성을 갖춘 인공지능('19)
- (美국립표준연구소, NIST) 인공지능이 사회 편익, 경제 성장을 위해 활용될 경우 반드시 만족시켜야 하는 목표이며, 설명가능성, 안전성, 보안성 등을 포함하는 개념('20)
- (유럽위원회, EC) 인공지능은 활용 및 동작이 합법적이며, 윤리적이고 기술적・사회적으로 견고해야 함('19)
3. 국내외 인공지능 신뢰성 정책 및 연구 동향
유럽위원회, 미국 등 주요국들은 인공지능의 신뢰성 확보가 인공지능의 사회적・산업적 수용과 발전의 전제 조건으로 정의하고 신뢰성 확보 정책을 추진하고 있다. 또한 산업계 및 학계에서도 관련 기술 개발을 중심으로 신뢰성 확보를 위한 연구가 활발하다. 구체적으로 유럽위원회, 미국 등 주요국에서는 인공지능 신뢰성을 확보하는 데 필요한 정책과 규범을 본격적으로 마련하고 있으며, 이와 함께 국가 차원의 인공지능 전략 핵심 요소로 Trustworthy AI, Safe AI 등을 명시했다. 특히, 유럽위원회는 지난 2021년도에 규제안을 제시하는 등 선제적으로 신뢰성 확보를 위한 법제화 움직임을 보이고 있다. 한편, 민간 부문에서는 인공지능 신뢰성 확보를 위한 가이드라인을 마련하여 자율적으로 인공지능의 신뢰성을 점검하고 확보할 수 있는 환경을 조성하고자 노력하고 있다. 기술 분야에서는 미국, 유럽 등 주요국의 학계와 글로벌 기업이 인공지능 신뢰성 확보에 필요한 제반 기술을 개발중이다. 우리나라도 글로벌 동향에 맞춰 "인공지능(AI) 윤리기준('20.12)", "신뢰할 수 있는 인공지능 실현 전략('21.5)"을 발표하며 정책 및 연구개발 양면에서 발 빠르게 움직이고 있다.
주요국 인공지능 신뢰성 관련 정책 동향
국가 | 주요 정책(연도) | 특징 |
---|---|---|
한국 |
|
사람 중심 인공지능을 기본가치로 인공지능 생태계 구축・인재양성・산업확산・역기능방지 등 종합 정책 추진 |
유럽위원회 |
|
인간중심의 가치, 윤리, 보안 등 균형 잡힌 인공지능 정책 추진 지향 |
유네스코 |
|
유네스코 회원국 193개국이 만장일치로 채택한 인공지능 윤리 국제 지침 |
미국 |
|
산업 분야별 인공지능 활용・촉진을 위한 인공지능 기술 개발 지원과 규제 완화 정책에 중점 |
중국 |
|
정부 주도의 대규모 투자와 강력한 인력양성, 데이터 개방・공유 등 기업친화적 정책 추진 |
일본 |
|
경제, 산업, 사회, 윤리 등의 관점에서 포괄적 접근 |
싱가포르 |
|
설명가능성・투명성・공정성, 인간 중심의 원칙에 기반하는 행동지침을 4가지 핵심영역별로 제시 |
해외 주요 산・학・연 인공지능 신뢰성 연구 동향
기관명 | 활동 및 내용 |
---|---|
美 방위고등연구계획국DARPA | 지능형 시스템에 대한 안전성.신뢰성 확보 연구(Assured Autonomy) 및 설명가능한 인공지능XAI, eXplainable AI 연구.개발 프로젝트 수행 중 |
美 국립표준기술연구소NIST | 글로벌 기업 및 연구기관과 공동으로 기업‧실무자가 활용 가능한 인공지능 위험관리 프레임워크Risk Management Framework 개발 중 |
美 스탠포드 대학 | 매년 인공지능 기술 수준 및 동향을 수록한 ‘AI Index’를 공개하고 있으며, 인공지능 안전성 관련 연구 수행 중 |
IBM | ‘Trusted AI’를 모토로, 공정성.설명가능성.강건성 확보를 위한 내부 업무 지침 및 백서, 개발.검증 도구 공개 |
Microsoft | ’Responsible AI’를 모토로, 공정성.설명가능성.투명성 확보를 위한 내부 지침 및 업무 표준, 개발.검증 도구 공개 |
‘Responsible AI’ 개발을 위한 원칙 제정, 신뢰성 확인 및 검증을 위한 가이드라인과 도구 공개 |
신뢰할 수 있는 인공지능 개발안내서 소개
국내외 많은 기관 및 기업이 인공지능 신뢰성 확보를 위한 윤리 원칙과 지침, 가이드라인을 내놓았으나, 기술적 관점에서 상세한 방법론을 제시한 사례는 아직 없었다. 따라서 인공지능 제품 및 서비스 개발 현장에서 데이터 과학자, 모델 개발자 등 이해관계자들이 실무 관점에서 신뢰성 확보를 위해 점검할 수 있는 항목을 개발하였으며 그 과정은 다음과 같다.
1. 개발 안내서 설계 요소(인공지능 서비스 구성, 생명주기, 신뢰성 요건)
개발 과정 중 가장 우선적으로 신뢰성 확보를 위해 어떤 요소들이 실무적으로 고려되어야 하는지 탐색해보았고, 그 결과 세 가지 설계 요소를 도출하여 요구사항 및 검증항목을 개발하였다. 각 설계 요소들은 요구사항과 검증항목 마련 시 모두 반영되었으며, 이러한 접근법을 아래 그림과 같이 매트릭스 형태로 체계화하여 ‘인공지능 신뢰성 프레임워크’로 정의하였다.
인공지능 신뢰성 프레임워크
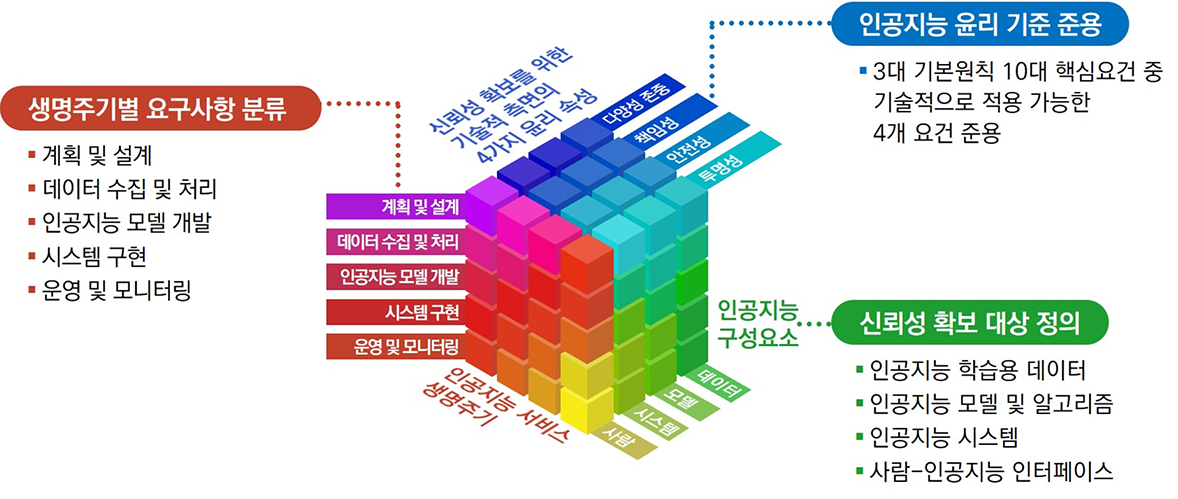
첫 번째는 인공지능 구성요소이다. 인공지능을 구성하는 4가지 요소는 인공지능 학습용 데이터, 학습과 추론 기능을 수행하는 인공지능 모델 및 알고리즘, 실제 기능을 구현할 시스템, 사용자와 상호작용하기 위한 인터페이스가 있다. 각 구성 요소들은 개별적으로 또는 통합적으로 인공지능 서비스의 생명주기에 따라 개발, 검증 및 운영된다. 따라서 구성요소별 신뢰성 확보 방안을 고민하고, 각 요소에 따른 요구사항과 검증항목을 제시하고자 했다. 각 요소에 대한 신뢰성 확보 방안은 다음과 같다.
인공지능 서비스 구성 요소
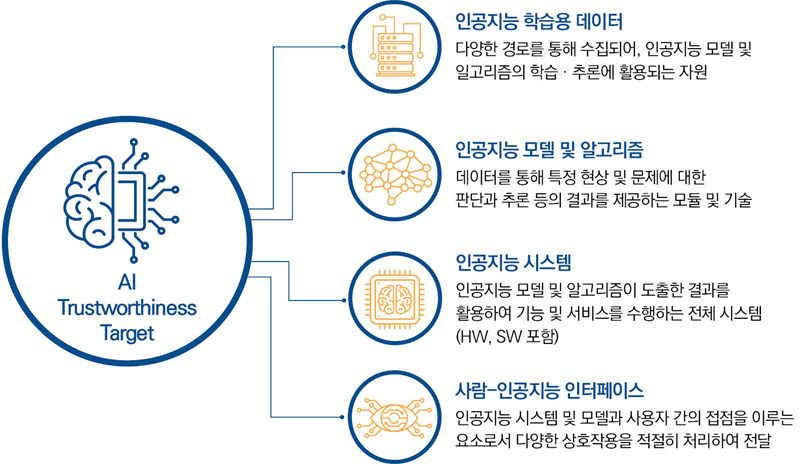
인공지능 서비스 구성 요소 | 신뢰성 확보 방안 |
---|---|
인공지능 학습용 데이터 | 인공지능 학습 및 추론 과정에 활용하는 데이터를 대상으로 편향성 및 공평성 등이 배제되었는지 검증 |
인공지능 모델 및 알고리즘 | 인공지능이 모델 및 알고리즘에 따라 안전한 결과를 도출하며, 이에 대한 설명이 가능한지, 악의적인 공격에 강건한지 등을 검증 |
인공지능 시스템 | 인공지능 모델 및 알고리즘이 적용된 전체 시스템을 대상으로 인공지능이 추론한 대로 작동하는지, 인공지능이 잘못 추론한 경우의 대책이 존재하는지 등을 검증 |
사람-인공지능 인터페이스 | 인공지능 시스템 사용자・운영자 등이 인공지능 시스템의 동작을 쉽게 이해할 수 있으며, 인공지능의 오작동 시 사람에게 알리거나 제어권을 이양하는지 등을 검증 |
두 번째, 인공지능 서비스 생명주기는 첫 번째에서 살펴본 인공지능 서비스 구성 요소들을 구현하고 운영하는 일련의 절차를 말한다. 기존 소프트웨어 시스템에서 다루는 공학 프로세스나 생명주기와 비슷하나, 인공지능 특성상 데이터 처리 및 모델 개발 단계가 별도로 필요하며, 이외의 단계에서도 주요 활동에 대한 정의가 조금씩 달라진다. 현재 인공지능 혹은 인공지능 서비스의 생명주기는 다수의 문헌에서 6~8가지 단계로 구분하고 있다. 대표적으로 OECD와 ISO/IEC에서 제시한 생명주기가 있는데, AI TrustOps에서는 두 기구에서 제시한 생명주기를 대표성 있는 사례로 참고하여, 실무자들이 쉽게 활용할 수 있도록 각 생명주기 단계의 성격과 활동을 왜곡하지 않는 선에서 아래와 같이 5가지 단계로 정리하였다.
인공지능 서비스 생명주기별 주요 활동
생명주기 단계 | 주요 활동 |
---|---|
1. 계획 및 설계 |
|
2. 데이터 수집 및 처리 |
|
3. 인공지능 모델 개발 |
|
4. 시스템 구현 |
|
5. 운영 및 모니터링 |
|
인공지능 서비스의 생명주기 단계는 반복적, 순환적인 성격을 갖지만, 반드시 순차적인 것은 아니다. 따라서, 이해를 돕기 위해 1단계부터 5단계까지 순차적인 것처럼 설명했으나, 실제 데이터를 수집하고 가공하거나 모델을 개발, 운영하는 과정에서는 순서가 달라질 수 있다.
세 번째, 인공지능 신뢰성에 필요한 요건을 정의하고자 '인공지능 윤리기준'의 10대 핵심요건을 준용하여 기술적 관점에서 필요한 요구사항과 검증항목으로 ‘다양성 존중’, ‘책임성’, ‘안전성’, ‘투명성’을 도출했다.
EC, OECD, IEEE 및 ISO/IEC와 같은 국제기구 등은 인공지능 신뢰성의 하위 속성들을 세분화해 제시하고 있다. 특히, ISO/IEC 24028:2020은 신뢰성 확보에 필요한 고려사항의 형태로 키워드를 제공한다. 여기에는 투명성, 통제가능성, 강건성, 복구성, 공평성, 안전성, 개인정보보호, 보안성 등이 포함되나, 키워드간의 관계나 신뢰성과의 연관성은 정의되지 않았다. 이처럼 관점에 따라 유사해 보이지만 조금씩 다른 용어들이 여러 문헌에서 제각각 달리 정의되고 있으며, 아직 합의된 속성 분류나 정의는 없는 상황이다. 이에, 앞서 언급한 EC, OECD, IEEE, ISO/IEC 등 여러 기구에서 제시한 속성과 키워드를 종합적으로 분석하고, 국내 학계.연구계.산업계 전문가의 의견을 수렴해 합의점을 모색했다. 이처럼 폭넓은 의견 공유 과정을 거쳐 인공지능 신뢰성 속성을 도출한 후, 이를 국가 인공지능 윤리기준의 10대 요건에 대응시켜서 기술적 측면에서 다룰만한 요건을 최종 선정하였다. 각 요건에 대한 정의는 아래와 같다.
인공지능 신뢰성 요건
신뢰성 요건 | 정의 |
---|---|
다양성 존중 | 인공지능이 특정 개인이나 그룹에 대한 차별적이고 편향된 관행을 학습하거나 결과를 출력하지 않으며, 인종・성별・연령 등과 같은 특성과 관계없이 모든 사람이 평등하게 인공지능 기술의 혜택을 받을 수 있는 것
|
책임성 | 인공지능이 생명주기 전반에 걸쳐 추론 결과에 대한 책임을 보장하기 위한 메커니즘이 마련되어 있는 것
|
안전성 |
|
투명성 | 인공지능이 추론한 결과를 인간이 이해하고 추적할 수 있으며, 인공지능이 추론한 결과임을 알 수 있는 것
|
위와 같이 인공지능 신뢰성 확보를 위한 다양한 속성들이 있으며, 각 신뢰성 속성들에 대한 정의를 파악하는 것뿐만 아니라 신뢰성 속성 간의 상호의존 관계 역시 중요하게 고려되어야 한다. 예를 들어, 인공지능 서비스에 대한 과도한 투명성 요구는 프라이버시 관련 위험을 초래할 수 있다. 또한, 설명가능성만으로는 투명성을 보장하기에 부족하지만, 설명가능성은 투명성을 확보하기 위한 중요한 요소 중 하나이다. 따라서, 인공지능 신뢰성 속성에 대한 충분한 이해를 바탕으로 인공지능 서비스를 제공하는 것이 중요하며, 해당 인공지능 서비스가 고려한 속성에 대해 적절하게 이행하고 는지 지속적으로 검토해야 한다.
2. '신뢰할 수 있는 인공지능 개발 안내서' 참고문헌
다음 단계로 구체적인 요구사항과 검증항목을 도출했다. 우선 표준화기구, 기술단체, 국제기구, 주요국에서 인공지능 신뢰성 확보를 위해 발표한 정책, 권고안, 그리고 표준을 기반으로 기술적 요구사항을 도출하고 구체화하였다. 이와 함께 AI 개인정보보호 자율점검표('21.5), 금융분야 AI 가이드라인('21.7) 등 국내에서 인공지능 신뢰성 확보를 목적으로 발표된 점검표 등을 검토했다. 검토 과정에서 개발 안내서에 필요한 내용은 반영하고 중복된 내용은 제거하거나 통합하였다. 참고문헌은 다음과 같다.
인공지능 신뢰성 관련 주요 참고문헌
기관명 | 발간년월 | 권고 및 표준안 명 |
---|---|---|
대한민국 | 2020.11 | 국가 인공지능(AI) 윤리기준 |
유럽위원회 | 2020.07 | The Assessment List for Trustworthy Artificial Intelligence |
유네스코 (UNESCO) |
2021.11 | Recommendation on The Ethics of Artificial Intelligence |
국제표준화기구 (ISO/IEC) |
2021.11 | ISO/IEC TR 24027:2021, Information technology - Artificial Intelligence (AI) - Bias in AI systems and AI aided decision making |
2021.03 | ISO/IEC TR 24029-1:2021, Artificial Intelligence (AI) - Assessment of the robustness of neural networks - Part 1: Overview | |
2021.01 | ISO/IEC 23894, Information Technology - Artificial Intelligence Risk Management (개발 중) | |
2020.05 | ISO/IEC TR 24028:2020,– AI - Overview of Trustworthiness in artificial intelligence | |
美 국립표준연구소 (NIST) |
2022.08 | Risk Management Framework: Second Draft |
세계경제포럼 (WEF) |
2020.01 | Companion to the Model AI Governance Framework |
경제협력개발기구 (OECD) |
2019.05 | Recommendation of the Council on Artificial Intelligence |
2019.05 | People + AI guidebook | |
유럽전기통신표준협회 (ETSI) |
2021.03 | Securing Artificial Intelligence (SAI 005) - Mitigation Strategy Report |